If you’re a fraud analyst or investigator using a graph database in your fraud detection process, this webinar is for you.
We’ll show how to visualize your data and create flexible, scalable and high-performance visual tools to make fraud detection faster, simpler and more robust.
The example here uses Amazon Neptune’s graph database, but our database-agnostic technology can visualize your data, no matter what datastore you prefer.
Graph visualization, graph databases and fraud detection
This webinar focuses on why graph databases and graph visualization should be at the core of your fraud detection program.
We start with an introduction to the Amazon Neptune graph database from Brad Bebee, General Manager at AWS. AWS built Amazon Neptune because they recognized how people increasingly wanted to build products that process highly-connected data that could answer questions about relationships. This need for a reliable graph database existed across vastly different use cases, including fraud detection.
Our data visualization toolkits are database-agnostic, so they integrate seamlessly with Amazon Neptune or any other datastore.
Many of our customers use our technology to help them detect and prevent fraud, and in this webinar, VP of Sales Christian Miles shares real-world examples of:
- Insurance fraud: many rely on alerts against insurance claims and policies to flag suspicious activities
- Credit card fraud: analysts can pick apart exactly what happened when suspected fraud took place, the geographical locations, which merchants were used, and more
- Review fraud: businesses treat their online review ratings as their IP and use data visualization to identify and eliminate fakes
Graph technologies play an important role in detecting fraud. When you pair our graph and timeline visualizations with an Amazon Neptune graph database to create a fraud detection tool, analysts and investigators can more easily discover insights and make decisions fast.
To demonstrate this, Christian visualizes NYC taxi cab data in light of certain news stories. They noticed an increase in the number of trips between workers at the Federal Reserve Bank of New York and major commercial banks, around the time central bank policy meetings were taking place. His demo also covers graph analysis workflows along with the techniques that drive effective fraud detection.
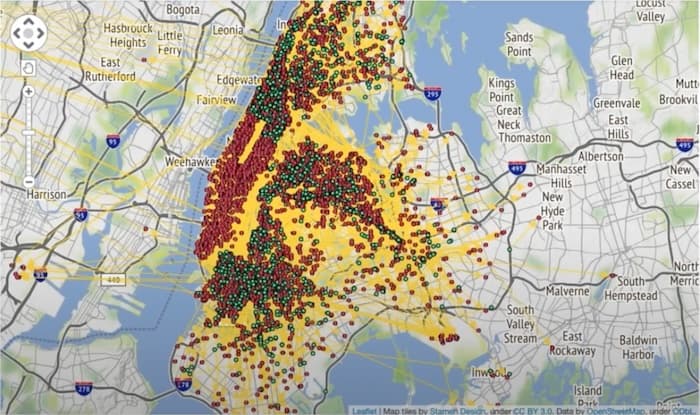